The transformative effects of machine learning and the onset of AI in healthcare systems around the world have huge implications for the improvement of our wellbeing. From better and faster diagnosis to understanding and managing symptoms to drug development, as well as the more efficient management of the systems themselves, the potential is almost endless.
AI has already woven itself into our lives through recommendations on social media as to what content we should click on and which adverts we see. AI systems utilise a user’s profile and demographic to make these decisions. Now, as its tools become increasingly applied to support healthcare, it may offer humanity more meaningful and lasting benefits.
Predictive power
Artificial Intelligence is a statistical software using algorithms that can find patterns in large amounts of data, whether words, images, video, audio, code, or even DNA, and then make predictions based upon these. This is different from the early days of computers, which were essentially based on a list of instructions similar to how calculators operate. Modern AI or machine-learning systems – ML being a subset of AI – predict what is the most likely outcome for something. As such, there is no definitive answer, but the technology considers what should come next based upon what it has seen in the past.
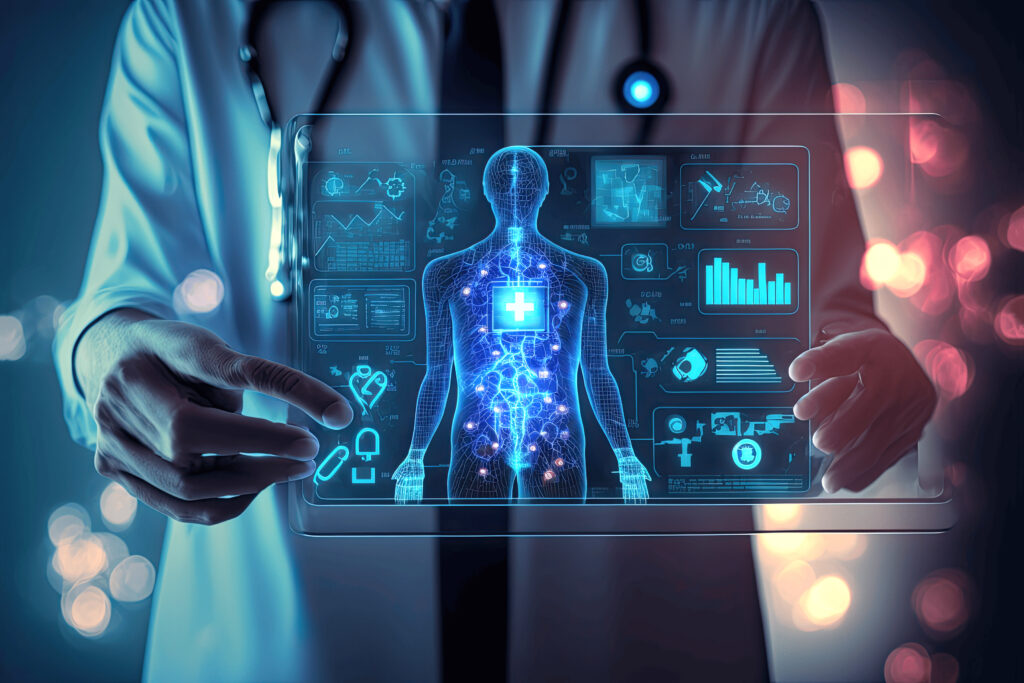
This formula is the building block for ChatGPT, which has the ability to find relationships between different words to predict which one should come next in a sentence, and ultimately compose advanced academic reports. The implication of this skill in making sense of big data from disparate sources is now becoming increasingly apparent in the provision of healthcare as medical research increasingly moves from code to clinic.
Success in sight
In ophthalmology, for example, AI is being used to prevent blindness. Pearse Keane, a Consultant Ophthalmologist at Moorfields Eye Hospital in the UK who specialises in the treatment of retinal disease and age-related macular degeneration, first considered the potential of artificial intelligence because patients were experiencing delays in getting an appointment with a doctor. Some, in critical cases, went blind as a result. He thought applying an AI system to data gleaned from retinal scans would help identify those whose sight was most under threat and enable them to receive attention within a few days rather than weeks or months.
He approached the co-founders of AI research laboratory DeepMind. “I told them we were doing 1,000 retinal scans per day at Moorfields and can we work together to develop an AI system to prevent blindness,” he relates in a BBC podcast. “The rest is history.”
Researchers at Moorfields shared about one million anonymised, historical scans with DeepMind and, essentially, trained AI algorithms to recognise eye disease. The performance of this powerful AI technology was “on par with world-leading experts from Moorfields for more than 50 different retinal diseases,” says Keane.
Window to the body
Interestingly, the retinal scans are also being considered for a new field called oculomics. The idea of using the eye as a window to the rest of the body to detect health conditions has been postulated for over a century. However, the ability to crunch and analyse vast amounts of data at speed has spawned a potential breakthrough. Keane enthuses: “What has been really exciting in the last four or five years is this idea that if you combine advanced retinal imaging with big data, with the latest advances in AI, it turns out that you can actually potentially predict Parkinson’s disease or dementia, or predict the risk of heart attack or stroke.”
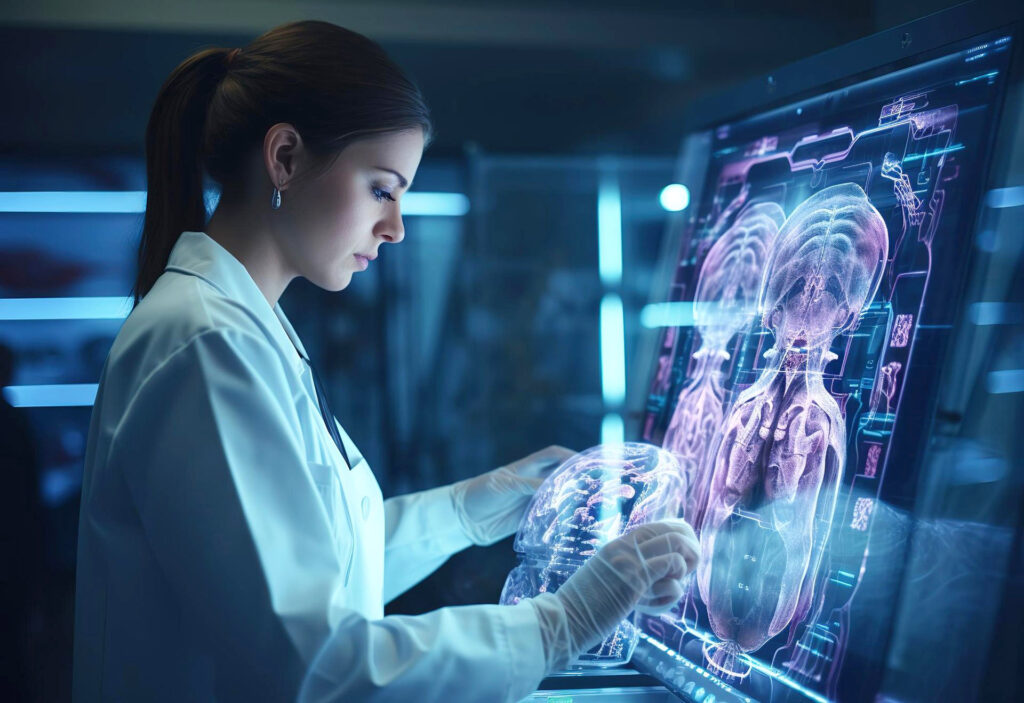
Although Keane is hopeful this technology will be widely available on an open-source basis within two or three years, he cautions: “But the caveat to that is we are still at the proof of concept; we are still pushing research-paper stage.”
Cancer detection tools
The life-saving potential of software trained to analyse millions and millions of scans was recently highlighted by an AI system called Mia used by the NHS in Scotland to detect signs of breast cancer that may be indiscernible to the human eye. Mia analysed the mammograms of more than 10,000 women and was able to flag all those with cancer, as well as an extra 11 the doctors did not identify. This ability to detect cancer at a much earlier stage could make the difference between survival and a potential early death, given that tumours can grow and spread very quickly.
Here in Hong Kong, researchers at the Hong Kong University of Science and Technology (HKUST) recently developed AI-based tools to detect 30 types of cancers and diseases. The university has developed four models which they believe are poised to transform the medical and healthcare fields, surpassing existing models due to their foundation on extensive data sets and novel machine-training strategies. Their breast-cancer diagnostic tool is said to achieve accuracy levels comparable to radiologists with five or more years of experience.
Another tool is poised to act like a “medical GPT”, with the ability to answer questions, generate medical reports, and provide initial diagnoses based on medical images. A further tool called XAIM (Explainable AI) is designed to enhance healthcare professionals’ confidence in adopting AI by explaining how medical AI models reach their decisions. Researchers at the university suggest that while many AI models offer high accuracy, they often lack transparency, and this leads to scepticism about their adoption.
“XAIM addresses this by providing visual and textual explanations of the models,” says a spokesperson for HKUST, which has previously developed an AI model predicting the prognosis of brain-tumour patients and a highly accurate blood test for early detection of Alzheimer’s disease.
Big data doubts
For sceptics, one of the issues with using AI in healthcare is that it relies on a vast data set and its output will necessarily reflect that data set and also any errors or specific biases it contains. For instance, marginalised populations who do not access healthcare will not be reflected in the data and the results will not therefore serve the population as a whole. Another concern is that models are perceived as having a “non-deterministic” nature, meaning they will not always respond in the same way and make the same predictions to the same stimuli. Privacy of personal data has also been flagged as an issue.
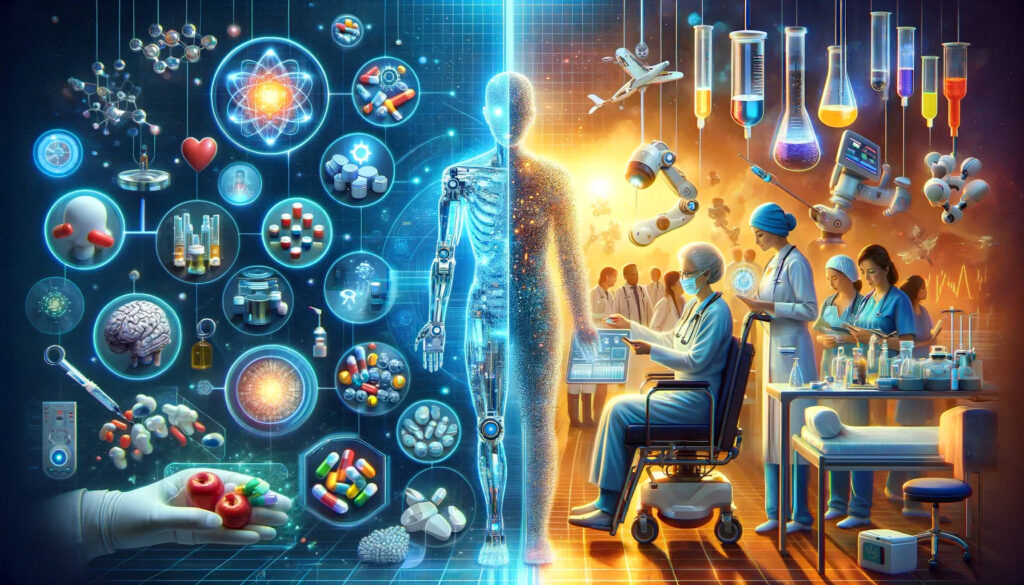
There is also the question of accountability. For instance, if an AI model is used to determine which patient receives a heart transplant and which does not, then who would be accountable if that judgment is subsequently proved to be wrong?
Dr Jessica Morley, a Postdoctoral Researcher at Yale University’s Digital Ethics Centre, believes current AI modelling lacks transparency, especially given the complexity of its development. She tells the BBC podcast this is the crux of the AI conundrum: “If we have come to the point where something the algorithm is making a “wrong” decision – where did the wrongness start? Is it who collected the data? Is it who curated it? So how did you decide what was cleaned and what was not? Is it who designed the model or who evaluated the model? Is it who interpreted the model?”
AI concerns and hopes
These sorts of ethical considerations are behind recent calls in Hong Kong for guardrails and protection to be put in place before the widespread rollout of AI in healthcare and other sectors, such as finance.
However, many in healthcare have high hopes that AI can also reap efficiency savings and increase productivity. Tools such as AI-backed voice transcriptions, for instance, could save a huge amount of time and cost for a health system. In 2022, a McKinsey report suggested using AI to predict diagnostics outcomes and support clinical decisions could create US$5 billion in economic value in China. Many poorer nations across Africa could also derive immense benefits from AI in healthcare.